Deep learning representations to support COVID-19 diagnosis on CT slices
Abstract
Introduction: The coronavirus disease 2019 (COVID-19) has become a significant public health problem worldwide. In this context, CT-scan automatic analysis has emerged as a COVID-19 complementary diagnosis tool allowing for radiological finding characterization, patient categorization, and disease follow-up. However, this analysis depends on the radiologist’s expertise, which may result in subjective evaluations.
Objective: To explore deep learning representations, trained from thoracic CT-slices, to automatically distinguish COVID-19 disease from control samples.
Materials and methods: Two datasets were used: SARS-CoV-2 CT Scan (Set-1) and FOSCAL clinic’s dataset (Set-2). The deep representations took advantage of supervised learning models previously trained on the natural image domain, which were adjusted following a transfer learning scheme. The deep classification was carried out: (a) via an end-to-end deep learning approach and (b) via random forest and support vector machine classifiers by feeding the deep representation embedding vectors into these classifiers.
Results: The end-to-end classification achieved an average accuracy of 92.33% (89.70% precision) for Set-1 and 96.99% (96.62% precision) for Set-2. The deep feature embedding with a support vector machine achieved an average accuracy of 91.40% (95.77% precision) and 96.00% (94.74% precision) for Set-1 and Set-2, respectively.
Conclusion: Deep representations have achieved outstanding performance in the identification of COVID-19 cases on CT scans demonstrating good characterization of the COVID-19 radiological patterns. These representations could potentially support the COVID-19 diagnosis in clinical settings.
Downloads
References
Guarner J. Three emerging coronaviruses in two decades: The story of SARS, MERS, and now COVID-19. Am J Clin Pathol. 2020;153:420-1. https://doi.org/10.1093/ajcp/aqaa029
Liu R, Han H, Liu F, Lv Z, Wu K, Liu Y, et al. Positive rate of RT-PCR detection of SARSCoV-2 infection in 4880 cases from one hospital in Wuhan, China, from Jan to Feb 2020. Clin Chim Acta. 2020;505:172-5. https://doi.org/10.1016/j.cca.2020.03.009
Johns Hopkins University & Medicine. COVID-19 Dashboard by the Center for Systems Science and Engineering (CSSE) at Johns Hopkins University (JHU). Accessed: June 5, 2021. Disponible en: https://coronavirus.jhu.edu/map.html
Wiersinga WJ, Rhodes A, Cheng AC, Peacock SJ, Prescott HC. Pathophysiology, transmission, diagnosis, and treatment of coronavirus disease 2019 (COVID-19): a review. JAMA. 2020;324:782-93. https://doi.org/10.1001/jama.2020.12839
Li L, Qin L, Xu Z, Yin Y, Wang X, Kong B, et al. Artificial intelligence distinguishes COVID-19 from community acquired pneumonia on chest CT. Radiology. 2020. https://doi.org/10.1148/radiol.2020200905
Aleta A, Martín-Corral D, Piontti AP, Ajelli M, Litvinova M, Chinazzi M, et al. Modelling the impact of testing, contact tracing and household quarantine on second waves of COVID-19. Nat Hum Behav. 2020;4:964-71. https://doi.org/10.1038/s41562-020-0931-9
Wang W, Xu Y, Gao R, Lu R, Han K, Wu G, et al. Detection of SARS-CoV-2 in different types of clinical specimens. JAMA. 2020;323:1843-4. https://doi.org/10.1001/jama.2020.3786
Kucirka LM, Lauer SA, Laeyendecker O, Boon D, Lessler J. Variation in false-negative rate of reverse transcriptase polymerase chain reaction–based SARS-CoV-2 tests by time since exposure. Ann Intern Med. 2020;173:262-7. https://doi.org/10.7326/M20-1495
Y. Kortela E, Kirjavainen V, Ahava MJ, Jokiranta ST, But A, Lindahl A, et al. Real-life clinical sensitivity of SARS-CoV-2 RT-PCR test in symptomatic patients. PLoS ONE. 2021;16:e0251661. https://doi.org/10.1371/journal.pone.0251661
Liang LL, Tseng CH, Ho HJ, Wu CY. Covid-19 mortality is negatively associated with test number and government effectiveness. Sci Rep. 2020;10:12567. https://doi.org/10.1038/s41598-020-68862-x
Inui S, Fujikawa A, Jitsu M, Kunishima N, Watanabe S, Suzuki Y, et al. Chest CT findings in cases from the cruise ship “Diamond Princess” with coronavirus disease 2019 (COVID-19). Radiol Cardiothorac Imaging. 2020;2:e200110. https://doi.org/10.1148/ryct.2020200110
Dai Wc, Zhang Hw, Yu J, Xu Hj, Chen H, Luo Sp, et al. CT imaging and differential diagnosis of COVID-19. Can Assoc Radiol J. 2020;71:195-200. https://doi.org/ 10.1177/0846537120913033
Fang Y, Zhang H, Xie J, Lin M, Ying L, Pang P, et al. Sensitivity of chest CT for COVID-19: comparison to RT-PCR. Radiology. 2020;296:E115-7. https://doi.org/10.1148/radiol.2020200432
Hope MD, Raptis CA, Shah A, Hammer MM, Henry TS. A role for CT in COVID-19? What data really tell us so far. Lancet. 2020;395:1189-90. https://doi.org/10.1016/S0140-6736(20)30728-5
Bai HX, Hsieh B, Xiong Z, Halsey K, Choi JW, Tran TML, et al. Performance of radiologists in differentiating COVID-19 from viral pneumonia on chest CT. Radiology. 2020;296:E46-54 https://doi.org/10.1148/radiol.2020200823
Xiao AT, Tong YX, Zhang S. False-negative of RT-PCR and prolonged nucleic acid conversion in COVID-19: Rather than recurrence. J Med Virol. 2020;92:1755-6. https://doi.org/10.1002/jmv.25855
Mahomed N, van Ginneken B, Philipsen RH, Meléndez J, Moore DP, Moodley H, et al. Computer-aided diagnosis for World Health Organization-defined chest radiograph primaryendpoint pneumonia in children. Pediatr Radiol. 2020;50:482-91. https://doi.org/10.1007/s00247-019-04593-0
Kermany DS, Goldbaum M, Cai W, Valentim CC, Liang H, Baxter SL, et al. Identifying medical diagnoses and treatable diseases by image-based deep learning. Cell. 2018;172:1122-31. https://doi.org/10.1016/j.cell.2018.02.010
Silva P, Luz E, Silva G, Moreira G, Silva R, Lucio D, et al. COVID-19 detection in CT images with deep learning: A voting-based scheme and cross-datasets analysis. Inform Med Unlocked. 2020;20:100427. https://doi.org/10.1016/j.imu.2020.100427
Ragab DA, Attallah O. FUSI-CAD: Coronavirus (COVID-19) diagnosis based on the fusion of CNNs and handcrafted features. PeerJ Comput Sci. 2020;6:e306. https://doi.org/10.7717/peerj-cs.306
Deng J, Dong W, Socher R, Li LJ, Li K, Fei-Fei L. ImageNet: A large-scale hierarchical image database. IEEE Conference on Computer Vision and Pattern Recognition. 2009. https://doi.org/10.1109/CVPR.2009.5206848
Soares E, Angelov P, Biaso S, Froes MH, Abe DK. SARS-CoV-2 CT-scan dataset: A large dataset of real patients CT scans for SARS-CoV-2 identification. medRxiv. 2020. https://doi.org/10.1101/2020.04.24.20078584
Hansell DM, Bankier AA, MacMahon H, McLoud TC, Muller NL, Remy J. Fleischner Society: Glossary of terms for thoracic imaging. Radiology. 2008;246:697-722. https://doi.org/10.1148/radiol.2462070712
Pan F, Ye T, Sun P, Gui S, Liang B, Li L, et al. Time course of lung changes on chest CT during recovery from 2019 novel coronavirus (COVID-19) pneumonia. Radiology. 2020;295:715-21. https://doi.org/10.1148/radiol.2020200370
Bernheim A, Mei X, Huang M, Yang Y, Fayad ZA, Zhang N, et al. Chest CT findings in coronavirus disease-19 (COVID-19): Relationship to duration of infection. Radiology. 2020;295:200463. https://doi.org/10.1148/radiol.2020200463
Parekh M, Donuru A, Balasubramanya R, Kapur S. Review of the chest CT differential diagnosis of ground-glass opacities in the COVID era. Radiology. 2020:297:E289-302. https://doi.org/10.1148/radiol.2020202504
Simonyan K, Zisserman A. Very deep convolutional networks for large-scale image recognition. arXiv preprint arXiv:14091556. The 3rd International Conference on Learning Representations, 2015. Disponible en: https://arxiv.org/abs/1409.1556
He K, Zhang X, Ren S, Sun J. Deep residual learning for image recognition. IEEE Conference on Computer Vision and Pattern Recognition. 2016. https://doi.org/10.1109/CVPR.2016.90
Szegedy C, Vanhoucke V, Ioffe S, Shlens J, Wojna Z. Rethinking the inception architecture for computer vision. IEEE Conference on Computer Vision and Pattern Recognition. 2016. https://doi.org/10.1109/CVPR.2016.308
Tan C, Sun F, Kong T, Zhang W, Yang C, Liu C. A survey on deep transfer learning. In: Kůrková V, Manolopoulos Y, Hammer B, Iliadis L, Maglogiannis I, editors. Artificial Neural Networks and Machine Learning – ICANN 2018. Lecture Notes in Computer Science, vol. 11141. Springer, Cham, Switzerland; 2018. https://doi.org/10.1007/978-3-030-01424-7_27
Hijazi S, Kumar R, Rowen C. Using convolutional neural networks for image recognition. San José, CA, USA: Cadence Design Systems Inc; 2015. p. 1-12.
Akilan T, Wu QJ, Jiang W. A feature embedding strategy for high-level CNN representations from multiple convnets. IEEE Global Conference on Signal and Information Processing. 2017. https://doi.org/10.1109/GlobalSIP.2017.8309150
Pham TD. A comprehensive study on classification of COVID-19 on computed tomography with pretrained convolutional neural networks. Sci Rep. 2020;10:1-8. https://doi.org/10.1038/s41598-020-74164-z
Alebiosu DO, Muhammad FP. Medical Image Classification: A Comparison of Deep Pretrained Neural Networks. IEEE Student Conference on Research and Development. 2019. https://doi.org/10.1109/SCORED.2019.8896277
Breiman L. Random forests. Machine Learning. 2001;45:5-32. https://doi.org/10.1023/A:1010933404324
Savas C, Dovis F. The impact of different kernel functions on the performance of scintillation detection based on support vector machines. Sensors. 2019;19:5219. https://doi.org/10.3390/s19235219
Amami R, Ayed DB, Ellouze N. Practical selection of SVM supervised parameters with different feature representations for vowel recognition. International Journal of Digital Content Technology and its Applications. 2013;7(9). https://doi.org/10.48550/arXiv.1507.06020
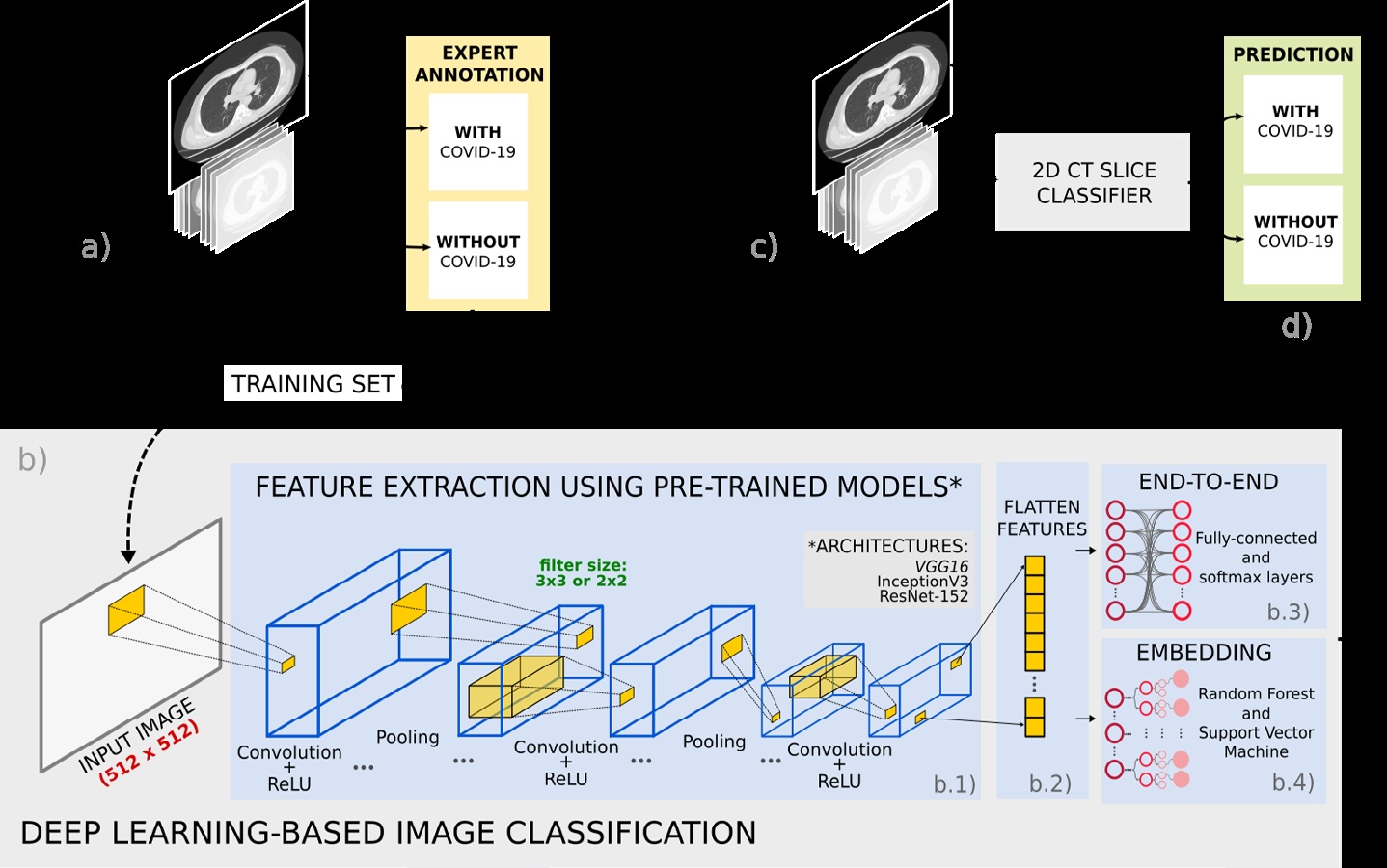
Article metrics | |
---|---|
Abstract views | |
Galley vies | |
PDF Views | |
HTML views | |
Other views |