Biogeographical factors determining Triatoma recurva distribution in Chihuahua, México, 2014
Abstract
Introduction: Triatoma recurva is a Trypanosoma cruzi vector whose distribution and biological development are determined by factors that may influence the transmission of trypanosomiasis to humans.
Objective: To identify the potential spatial distribution of Triatoma recurve, as well as social factors determining its presence.
Materials and methods: We used the MaxEnt software to construct ecological niche models while bioclimatic variables (WorldClim) were derived from the monthly values of temperature and precipitation to generate biologically significant variables. The resulting cartography was interpreted as suitable areas for T. recurva presence.
Results: Our results showed that the precipitation during the driest month (Bio 14), the maximum temperature during the warmest month (Bio 5), and the altitude (Alt) and mean temperature during the driest quarter (Bio 9) determined T. recurva distribution area at a higher percentage evidencing its strong relationship with domestic and surrounding structures.
Conclusions. This methodology can be used in other geographical contexts to locate potential sampling sites where these triatomines occur.
Downloads
References
Vidal-Acosta V, Ibáñez-Bernal S, Martínez-Campos C. Infección natural de chinches Triatominae con Trypanosoma cruzi asociadas a la vivienda humana en México. Salud Pública de México. 2000;42:496-503.
Castro T, Hernández-Betancourt S, Puerto FI, Torres-León M. Lesiones histológicas asociadas a la posible infección por Trypanosoma cruzi (Chagas, 1909) en corazones de roedores sinantrópicos capturados en Yucatán, México. An Biol. 2016;38:29-35. https://doi.org/10.6018/analesbio.38.03
Medone P. Biogeografía de los insectos vectores de la enfermedad de Chagas: influencia de las variables ambientales sobre la distribución de los triatominos en América (tesis). Accessed: October 6, 2018. Available at: http://sedici.unlp.edu.ar/handle/10915/52775
Rivas N, Sánchez-Espíndola E, Camacho AD, Alejandre-Aguilar R. Comparative egg morphology of six Meccus species and Triatoma recurva (Stål, 1868) Hemiptera: Reduviidae. J Vector Ecol. 2016;41:135-41. https://doi.org/10.1111/jvec.12205
Padilla-Narváez FA. Ciclo de vida, hábitos de alimentación y defecación de Triatoma carrioni (Larrouse, 1926) pertenecientes a la provincia de Loja, bajo condiciones controladas de laboratorio. Accessed: October 6, 2018. Available at: http://repositorio.puce.edu.ec/handle/22000/10386
World Health Organization. The world health report 2002: Reducing risks, promoting healthy life. Accessed: October 6, 2018. Available at: https://www.who.int/whr/2002/en/
Nash D, Mostashari F, Fine A, Miller J, O’leary D, Murray K, et al. The outbreak of West Nile virus infection in the New York City area in 1999. New Engl J Med. 2001;344:1807-14. https://doi.org/10.1056/NEJM200106143442401
Ramírez-Sierra MJ, Dumonteil E. Infection rate by Trypanosoma cruzi and biased vertebrate host selection in the Triatoma dimidiata (Hemiptera: Reduvidae) species complex. J Med Entomol. 2016;53:20-5. https://doi.org/10.1093/jme/tjv168
Montenegro D, Vera M, Zuleta L, Llanos V, Junqueira A. Estrategia para determinar la línea base en áreas de interrupción vectorial de la enfermedad de Chagas. Rev Panam Salud Pública. 2016;39:341-51
Monroy ÁL, Pedraza AM, Prada CF. Prevalence of anti-Trypanosoma cruzi antibodies in women of childbearing age in Socotá, Boyacá, 2014. Biomédica. 2016;36:90-6. https://.doi.org/10.7705/biomedica.v36i3.2923
Ramsey J, Ordóñez R, Tello-López A, Pohls J, Sánchez V, Peterson A. Actualidades sobre la epidemiología de la enfermedad de Chagas en México. México: Instituto Nacional de Salud Pública; 2003. p. 85-104.
Parra-Henao G, Quirós-Gómez O, Jaramillo-O N, Cardona ÁS. Environmental determinants of the distribution of Chagas disease vector Triatoma dimidiata in Colombia. Am J Trop Med Hyg. 2016;94:767-74. https://doi.org/10.4269/ajtmh.15-0197
Cajo DJ, Moreno M, Chaguamate L, Valencia N, Ayala VR. Aplicación de modelos de nicho ecológico para estudios epidemiológicos: Triatoma dimidiata, vector de la enfermedad de Chagas en Ecuador. Revista Politécnica. 2016;37:88:92.
Marín E, Exébio C, Santillán R, Cuba C, Jurberg J, Galvão C. Observaciones de campo y algunos parámetros biológicos de los triatominae (Hemiptera: Reduviidae), vectores de la enfermedad de Chagas en el noriente de Perú. Pueblo Continente. 2014;25:53-74.
Morris E, Bone C. Identifying spatial data availability and spatial data needs for Chagas disease mitigation in South America. Spat Spatiotemporal Epidemiol. 2016;17:45-58. https://doi.org/10.1016/j.sste.2016.04.006
Gentry J, Sturm B, Peterson AT. Predictive mapping of transmission risk of a soil-transmitted helminth across East Africa: Findings from community prevalence surveys. J Public Health Dev Ctries. 2016;2:150-61.
Quinde-Calderón L, Ríos-Quituizaca P, Solorzano L, Dumonteil E. Ten years (2004–2014) of Chagas disease surveillance and vector control in Ecuador: Successes and challenges. Trop Med Int Health. 2016;21:84-92. https://doi.org/10.1111/tmi.12620
Urbina-Cardona JN, Loyola RD. Applying niche-based models to predict endangered-Hylid potential distributions: Are neotropical protected areas effective enough? Trop Conserv Sci. 2008;1:417-45. https://doi.org/10.1177/194008290800100408
INEGI. Territorio, población y economía de México, 2010. Accessed: August 25, 2018. Available at: http://www3.inegi.org.mx/sistemas/spc/Default.aspx
Hijmans RJ, Cameron SE, Parra JL, Jones PG, Jarvis A. Very high resolution interpolated climate surfaces for global land areas. Int J Climatol. 2005;25:1965-78. https://doi.org/10.1002/joc.1276
Young N, Carter L, Evangelista P. A MaxEnt model v3. 3.3 e tutorial (ArcGIS v10). Accessed: August 20, 2018. Available at: https://www.coloradoview.org/wp-content/coloradoviewData/trainingData/a-maxent-model-v8.pdf
Kawashima RS, Siqueira MF, Mantovani JE. Dados do monitoramento da cobertura vegetal por NDVI na modelagem da distribuição geográfica potencial do lobo-guará (Chrysocyon bracyurus). Florianópolis, Brasil: INPE; 2017. p. 3983-90.
Cruz-Cárdenas G, Villaseñor JL, López-Mata L, Martínez-Meyer E, Ortiz E. Selección de predictores ambientales para el modelado de la distribución de especies en Maxent. Rev Chapingo Ser Cie. 2014;20:187-201. https://doi.org/10.5154/r.rchscfa.2013.09.034
García E. Modificaciones al sistema de clasificación climática de Köppen. 5th edition. México D.F.: Instituto de Geografía UNAM; 2004.
González Lozano FA. Manual introductorio ArcGis 10.2. Accessed: August 20, 2018. Available at: http://bdigital.unal.edu.co/50837/1/manualintroductorioArcGis10.2.pdf
Torres R, Jayat JP. Modelos predictivos de distribución para cuatro especies de mamíferos (Cingulata, Artiodactyla y Rodentia) típicas del Chaco en Argentina. Mastozool Neotrop. 2010;17:335-52.
Martínez-Ortega RM, Tuya-Pendás LC, Martínez-Ortega M, Pérez-Abreu A, Cánovas AM. El coeficiente de correlación de los rangos de Spearman, caracterización. Revista Habanera de Ciencias Médicas. 2009;8:5.
Austin PC, Tu JV. Bootstrap methods for developing predictive models. Am Stat. 2004;58:131-7. https://doi.org/10.1198/0003130043277
Quiñones UM, Valerio PD, Ramos JH, Sánchez AM, Magaña JJ, Granados MC. Similaridad del nicho ecológico de Pinus montezumae y P. pseudostrobus (Pinaceae) en México: implicaciones para la selección de áreas productoras de semillas y de conservación. Acta Bot Mex. 2019. https://doi.org/10.21829/abm126.2019.1398
Elith J, Graham CH, Anderson RP, Dudík M, Ferrier S, Guisan A, et al. Novel methods improve prediction of species’ distributions from occurrence data. Ecography. 2006;29:129-51. https://doi.org/10.1111/j.2006.0906-7590.04596.x
Phillips SJ, Anderson RP, Schapire RE. Maximum entropy modeling of species geographic distributions. Ecol Model. 2006;190:231-59. https://doi.org/10.1016/j.ecolmodel.2005.03.026
Riverón-Giró FB, Raventós J, Damon A, García-González A, Mújica E. Spatio-temporal dynamics of the invasive orchid Oeceoclades maculata (Orchidaceae), in four different habitats in southeast Chiapas, Mexico. Biol Invasions. 2019;21:1905-19. https://doi.org/10.1007/s10530-019-01945-7
Duque PL, Liria J, Burgaleta E, Salazar J, Arrivillaga-Henríquez J, Enríquez S, et al. High mosquito diversity in an Amazonian village of Ecuador, surrounded by a Biological Reserve, using a rapid assessment method. J Entomol Acarological Res. 2019;51. https://doi.org/10.4081/jear.2019.7775
Pearson RG, Raxworthy CJ, Nakamura M, Townsend-Peterson A. Predicting species distributions from small numbers of occurrence records: a test case using cryptic geckos in Madagascar. J Biogeogr. 2007;34:102-17. https://doi.org/10.1111/j.1365-2699.2006.01594.x
Liu C, Newell G, White M. The effect of sample size on the accuracy of species distribution models: Considering both presences and pseudo-absences or background sites. Ecography. 2019;42:535-48. https://doi.org/10.1111/ecog.03188
Lyon NJ, Debinski DM, Rangwala I. Evaluating the utility of species distribution models in informing climate change-resilient grassland restoration strategy. Fron Ecol Evol. 2019;7:33. https://doi.org/10.3389/fevo.2019.00033
Fletcher Jr RJ, Hefley TJ, Robertson EP, Zuckerberg B, McCleery RA, Dorazio RM. A practical guide for combining data to model species distributions. Ecology. 2019;100. https://doi.org/10.1002/ecy.2710
Støa B, Halvorsen R, Stokland JN, Gusarov VI. How much is enough? Influence of number of presence observations on the performance of species distribution models. Sommerfeltia. 2019;39:1-28. https://doi.org/10.2478/som-2019-0001
Abdelaal M, Fois M, Fenu G, Bacchetta G. Using MaxEnt modeling to predict the potential distribution of the endemic plant Rosa arabica Crép. in Egypt. Ecol Inform. 2019;50:68-75. https://doi.org/10.1016/j.ecoinf.2019.01.003
Kariminejad N, Hosseinalizadeh M, Pourghasemi HR, Bernatek-Jakiel A, Campetella G, Ownegh M. Evaluation of factors affecting gully headcut location using summary statistics and the maximum entropy model: Golestan Province, NE Iran. Sci Total Environ. 2019;677:281-98. https://doi.org/10.1016/j.scitotenv.2019.04.306
Chen X, Dimitrov NB, Meyers LA. Uncertainty analysis of species distribution models. PLOS ONE. 2019;14:1-11. https://doi.org/10.1371/journal.pone.0214190
Fielding AH, Bell JF. A review of methods for the assessment of prediction errors in conservation presence/absence models. Environ Conserv. 1997;24:38-49. https://doi.org/10.1017/S0376892997000088
Guisan A, Broennimann O, Engler R, Vust M, Yoccoz NG, Lehmann A, et al. Using nichebased models to improve the sampling of rare species. Conserv Biol. 2006;20:501-11. https://doi.org/10.1111/j.1523-1739.2006.00354.x
Naranjo M, Moreno ÁC, Martín S. ¿Dónde buscar troglobiontes? Ensayo de una cartografía predictiva con MaxEnt en Gran Canaria (islas Canarias). Arxius de Miscellània Zoològica. 2014;12:83-92. https://doi.org/10.32800/amz.2014.12.0083
Comisión Nacional para la Biodiversidad. Portal de geoinformación, Sistema Nacional de Información Sobre Biodiversidad. Accessed: August 12, 2018. Available at: http://www.conabio.gob.mx/informacion/gis/
Waleckx E, Cámara-Mejía J, Ramírez-Sierra MJ, Cruz-Chan V, Rosado-Vallado M, Vázquez-Narváez S, et al. Una intervención innovadora de ecosalud para el control vectorial de la enfermedad de Chagas en Yucatán, México. Revista Biomédica. 2015;26:75-86.
Peña J, Perdomo LM, Cuartas DE. Geografía de la Salud, una visión de pasado y presente. Entorno Geográfico. 2013;9:146-58.
Chaves M, Esteban C, Gómez-Mantilla MA, Ballesteros-Orduz MF, Rangel-Laguado MA, Concha-Sánchez JG. Manual de modelamiento de datos geoespaciales para la identificación de la vocación agrológica de los predios del municipio de Piedecuesta según el dato del IGAC para el cultivo de la mora. Accessed: August 12, 2018. Available at: http://repositorio.uts.edu.co:8080/xmlui/handle/123456789/7821
Lorenzo MG, Guarneri AA, Pires HH, Diotaiuti L, Lazzari CR. Microclimatic properties of the Triatoma brasiliensis habitat. Cad Saúde Pública. 2000;16:S69-S74. https://doi.org/10.1590/S0102-311X2000000800007
Carbajal-de la Fuente AL, Fernández MP, Piccinali RV, Rodríguez-Planes LI, Duarte R, Gürtler RE. Occurrence of domestic and intrusive triatomines (Hemiptera: Reduviidae) in sylvatic habitats of the temperate Monte Desert ecoregion of Argentina. Acta Trop. 2019;196:37-41. https://doi.org/10.1016/j.actatropica.2019.04.028
Guarneri A, Diotaiuti L, Lazzari C, Lorenzo MG. The effect of relative humidity on Triatoma brasiliensis: Hygropreference and eclosion success. Mem Inst Oswaldo Cruz. 1998;93:349.
Parra-Henao G, Suárez-Escudero LC, González-Caro S. Potential distribution of Chagas disease vectors (Hemiptera: Reduviidae: Triatominae) in Colombia, based on ecological niche modeling. J Trop Med. 2016;2016:1-10. https://doi.org/10.1155/2016/1439090
Obara MT, da Silva Cardoso A, Pinto MC, de Souza CR, Silva RA, Gurgel-Gonçalves R. Eratyrus mucronatus Stål, 1859 (Hemiptera: Reduviidae: Triatominae): first report in the State of Acre, Brazil, and updated geographic distribution in South America. Check List. 2013;9:851-4. https://doi.org/10.15560/9.4.851
Di Luciano VS. Orientation of Triatoma infestans (Hemiptera: Reduviidae) to environmental temperatures. J Med Entomol. 1983;20:446-54.
Balsalobre A. ¿Qué especies de vinchucas modificarán su distribución geográfica en la Argentina? Accessed: August 21, 2018. Available at: http://sedici.unlp.edu.ar/handle/10915/52319
Martínez-Ibarra JA, Paredes-González E, Montañez-Valdez OD, Rocha-Chávez G, Nogueda-Torres B. La biología de tres especies mexicoamericanas de Triatominae (Hemiptera: Reduviidae): Triatoma recurva, Triatoma protracta y Triatoma rubida. Mem Inst Oswaldo Cruz. 2012;107:659-63. https://doi.org/10.1590/S0074-02762012000500013
Anderson RP, Lew D, Peterson AT. Evaluating predictive models of species’ distributions: Criteria for selecting optimal models. 2003; Ecological Modelling 162:211-32. https://doi.org/10.1016/S0304-3800(02)00349-6
Paredes-García DM, Ramírez-Bautista A, Martínez-Morales MA. Distribución y representatividad de las especies del género Crotalus en las áreas naturales protegidas de México. Revista Mexicana de Biodiversidad. 2011;82:689-700.
Some similar items:
- Marlene Reyes, Víctor Manuel Angulo, Life cycle of Triatoma dimidiata Latreille, 1811 (Hemiptera, Reduviidae) under laboratory conditions: production of nymphs for biological tests , Biomedica: Vol. 29 No. 1 (2009)
- Lyda Esteban, José Mauricio Montes, Víctor Manuel Angulo, Diversity of Triatominae (Hemiptera: Reduviidae) in Santander, Colombia: Epidemiological implications , Biomedica: Vol. 37 No. 1 (2017)
- Luis Alberto Corté, Henry Alberto Suárez, Triatomines (Reduviidae: Triatominae) in a Chagas disease focus in Talaigua Nuevo (Bolívar, Colombia). , Biomedica: Vol. 25 No. 4 (2005)
- Jaime Badel-Mogollón, Laura Rodríguez-Figueroa, Gabriel Parra-Henao, Spatio-temporal analysis of the biophysical and ecological conditions of Triatoma dimidiata (Hemiptera: Reduviidae: Triatominae) in the northeast region of Colombia , Biomedica: Vol. 37 No. Sup. 2 (2017): Suplemento 2, Entomología médica, 2017
- Luis Reinel Vásquez, Cleber Galvão, Néstor A. Pinto, Humberto Granados, First report of Triatoma nigromaculata (Stål, 1859) (Hemiptera, Reduviidae, Triatominae) for Colombia. , Biomedica: Vol. 25 No. 3 (2005)
- José Alejandro Martínez-Ibarra, Jorge Alejandro Martínez-Grant, Miguel Roberto Verdugo-Cervantes, Rafael Bustos-Saldaña, Benjamín Nogueda-Torres, Monitoring triatomid bug (Hemiptera: Reduviidae) presence by sentinel chicken coops in Southern Jalisco State, México , Biomedica: Vol. 30 No. 1 (2010)
- Nelson Grisales, Omar Triana, Víctor Angulo, Nicolás Jaramillo, Gabriel Parra-Henao, Francisco Panzera, Andrés Gómez-Palacio, Genetic differentiation of three Colombian populations of Triatoma dimidiata (Heteroptera: Reduviidae) by ND4 mitochondrial gene molecular analysis , Biomedica: Vol. 30 No. 2 (2010)
- Omar Cantillo-Barraza, Andrés Gómez-Palacio, Diego Salazar, Ana María Mejía-Jaramillo, Jaime Calle, Omar Triana, Distribution and ecoepidemiology of the triatomine fauna (Hemiptera: Reduviidae) in Margarita Island, Bolívar, Colombia , Biomedica: Vol. 30 No. 3 (2010)
- Marlene Reyes, Víctor Manuel Angulo, Claudia Magaly Sandoval, Toxic effect of b-cipermethrin, deltamethrin and fenitrothion in colonies of Triatoma dimidiata (Latreille, 1811) and Triatoma maculata (Erichson, 1848) (Hemiptera, Reduviidae) , Biomedica: Vol. 27 No. 1esp (2007): Enfermedad de Chagas
- Juan Carlos Quintero, María L. Félix, José M. Venzal , Santiago Nava, Ixodes tropicalis (Acari: Ixodidae) infesting a human and molecular detection of Rickettsia bellii, Colombia , Biomedica: Vol. 41 No. 2 (2021)
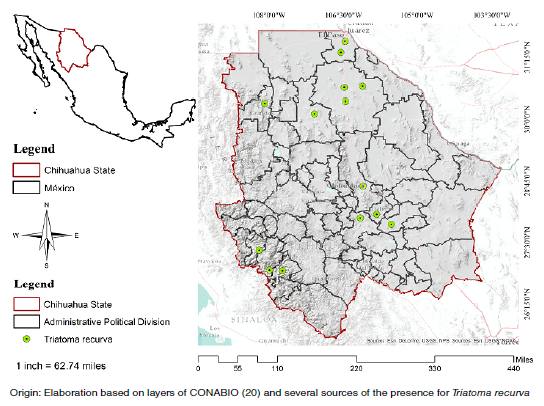
Article metrics | |
---|---|
Abstract views | |
Galley vies | |
PDF Views | |
HTML views | |
Other views |